I had the chance to take part within the Inaugural AI Business Lifecycle and Procurement Summit 2024 hosted by Curshaw. This was a really fascinating ‘unconference’ the place individuals supplied to guide periods on matters they wished to speak about. I led a session on ‘Creating friction in AI procurement’.
This was clearly a counterintuitive mind-set about AI and procurement, on condition that the ‘massive promise’ of AI is that it’s going to cut back friction (eg by way of automation, and/or delegation of ‘non-value-added’ duties). Why would I wish to create friction on this context?
The primary clarification I used to be thus requested for was whether or not this was about ‘good friction’ (versus outdated dangerous ‘crimson tape’ type of friction), which in fact it was (?!), and the second, what do I imply by friction.
My latest analysis on AI procurement (eg right here and right here for the book-long remedy) has led me to conclude that we have to decelerate the method of public sector AI adoption and to create mechanisms that convey again to the desk the ‘non-AI’ possibility and a number of other ‘cease venture’ or ‘deal breaker’ trumps to push again towards the tidal wave of unavoidability that appears to dominate all discussions on public sector digitalisation. My most well-liked resolution is to take action by way of a system of permissioning or licencing administered by an impartial authority—however I’m conscious and prepared to concede that there isn’t a political will for it. I thus began excited about second-best approaches to slowing public sector AI procurement. That is how I received to the thought of friction.
By creating friction, I imply the necessity for a structured decision-making course of that enables for collective deliberation inside and across the adopting establishment, and which is supported by rigorous influence assessments that tease out second and third order implications from AI adoption, in addition to completely interrogating first order points round knowledge high quality and governance, technological governance and organisational functionality, particularly round danger administration and mitigation. That is complementary—however hopefully goes past—rising frameworks to find out organisational ‘danger urge for food’ for AI procurement, akin to that developed by the AI Procurement Lab and the Centre for Inclusive Change.
The conversations the concentrate on ‘good friction’ moved in numerous instructions, however there are some takeaways and concepts that caught with me (or I managed to jot down in my notes whereas chatting to others), akin to (in no explicit order of significance or potential):
the potential for ‘AI minimisation’ or ‘non-AI equivalence’ to check the necessity for (particular) AI options—for those who can sufficiently approximate, or replicate, the identical useful consequence with out AI, or with a less complicated sort of AI, why not do it that manner?;
the necessity for a structured catalogue of options (and elements of options) which can be already accessible (generally in open entry, the place there may be a number of duplication) to tell such issues;
the significance of asking whether or not procuring AI is pushed by issues akin to availability of funding (is that this funded if completed with AI however not funded, or exhausting to fund on the similar stage, if completed in different methods?), which may clearly skew decision-making—the significance of contemplating the consequences of ‘digital industrial coverage’ on decision-making;
the facility (and relevance) of the deceptively easy query ‘is there an interdisciplinary staff to be devoted to this, and solely to this’?;
the significance of data and understanding of the tech and its implications from the start, and of experience within the translation of technical and governance necessities into procurement necessities, to keep away from ‘video games of likelihood’ whereby using ‘fashionable phrases’ (akin to ‘agile’ or ‘accountable’) might or might not result in the award of the contract to the best-placed and best-fitting (tech) supplier;
the likelihood to adapt civic monitoring or social witnessing mechanisms utilized in different contexts, akin to giant infrastructure initiatives, to be embedded in contract efficiency and auditing phases;
the significance of understanding displacement results and whether or not deploying an answer (AI or automation, or comparable) to take care of a bottleneck will merely displace the problem to a different (new) bottleneck someplace alongside the method;
the significance of understanding the broader organisational modifications required to seize the hoped for (productiveness) positive factors arising from the tech deployment;
the significance of fastidiously contemplating and resourcing the a lot wanted engagement of the ‘clever particular person’ that should verify the design and outputs of the AI, together with frontline staff and people on the receiving finish of the related choices or processes and the affected communities—the significance of making significant and efficient deliberative engagement mechanisms;
relatedly, the necessity to guarantee organisational engagement and alignment at each stage and each step of the AI (pre)procurement course of (on which I’d suggest studying this latest piece by Kawakami and colleagues);
the necessity to assess the impacts of modifications in scale, complexity, and error publicity;
the necessity to create sufficient circuit-breakers all through the method.
Definitely tons to mirror on and attempt to embed in future analysis and outreach efforts. Due to all those that participated within the dialog, and to these desirous about becoming a member of it. A structured manner to take action is thru this LinkedIn group.
#Creating #constructive #friction #procurement #Crack #Nut
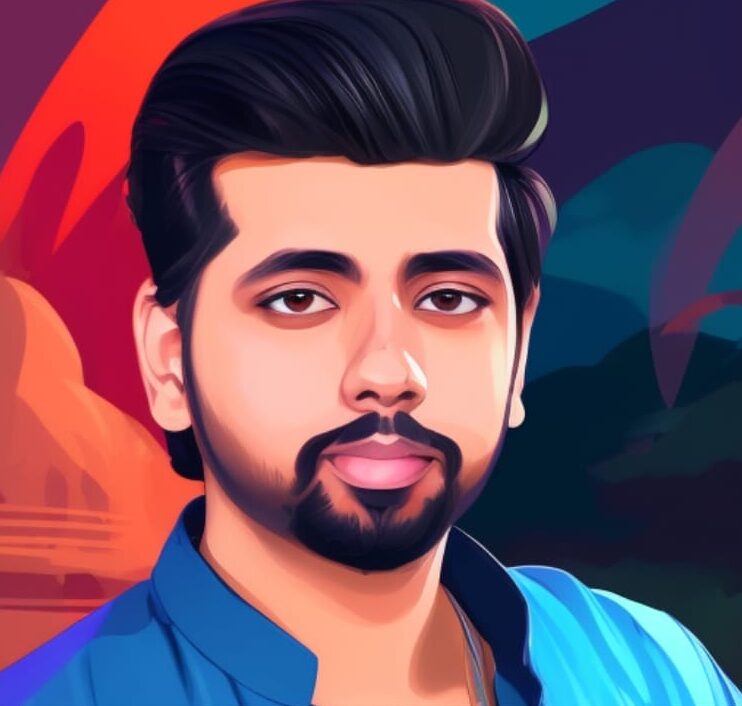
Azeem Rajpoot, the author behind This Blog, is a passionate tech enthusiast with a keen interest in exploring and sharing insights about the rapidly evolving world of technology.
With a background in Blogging, Azeem Rajpoot brings a unique perspective to the blog, offering in-depth analyses, reviews, and thought-provoking articles. Committed to making technology accessible to all, Azeem strives to deliver content that not only keeps readers informed about the latest trends but also sparks curiosity and discussions.
Follow Azeem on this exciting tech journey to stay updated and inspired.